The methodology behind our Claims Test tool is based on a proven choice-based technique & was refined through multiple projects for FMCG brands. Learn more here!
Claims Test
Efficiently test up to 300 product claims on customer appeal, fit with brand, and diagnostic questions of your choice.
Our Claims Test tool can help you test different product claims and messages. The methodology behind it is based on a proven choice-based technique and was refined through multiple projects for FMCG brands. In this post, we detail how the method works and why we prefer it over MaxDiff or an array of rating scale questions. Specifically we look into:
- Overall flow of questionnaire
- Comparison with MaxDiff
- Benefits of adaptive experimental design
- Allowing for cross-country comparisons
- Correlation data comparison
- Calculating Claims Test scores
Overall flow of questionnaire
The motivation behind the Claims Test tool is not only to make it easier for you to test multiple potential product claims, but also to:
- Introduce greater rigour into claims testing through a comprehensive, varied questionnaire
- Improve respondent experience compared to existing methods
- Allow for cross-country comparison of results
- Reduce sample costs
The quest to find an optimal versatile questionnaire flow led us to the following solution:
- Respondents see several screens where they choose the most motivating claim among several options (just like in conjoint)
- Then we look at several (four or so) claims separately, with the following screens for each claim:
- Reminder of claim
- Several diagnostic questions (with some questions shown in the positive, some in the negative)
- Choice of most relevant brand
- Open-ended feedback
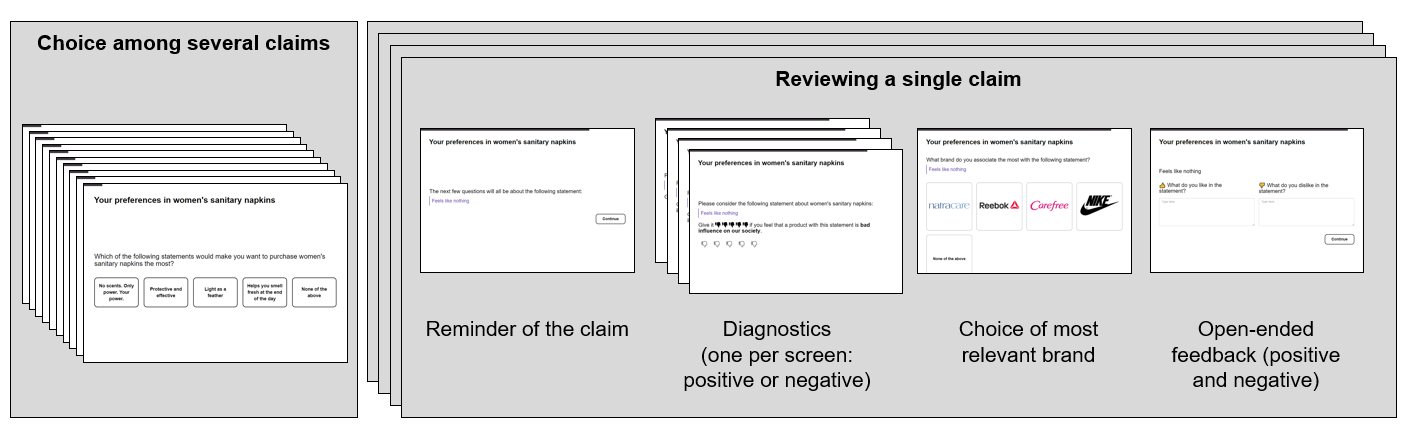
Why not MaxDiff?
MaxDiff will work fine in many cases, though in our experience MaxDiff has a few shortcomings that led us to developing the Claims Test:
- It is not as mobile-friendly as choice-based questionnaire. We tested multiple layouts offered by different software options and heard the same feedback from respondents: it is hard to view and select options on small screens.
- MaxDiff is not a natural task for people to do. Nobody goes shopping to pick the “best” and “worst”. When people shop, they select options they want to buy (or leave the shop if no option suits them). A choice-based exercise is a more realistic approximation of people’s real-life behaviour than a best-worst scale.
- MaxDiff’s focus on “worst” options does not address our clients’ needs: you want to know the best options for your product and “how good is good” rather than “how bad is bad”. It is not good use of respondents’ time (and your budget) to have people think about worst options.
The use of choice-based questions helped us build a simple and elegant respondent interface for optimal respondent experience and use the same robust quality checks to disqualify low-quality respondents as for conjoint analysis.
Another benefit of a choice-based approach is the presence of a “None of the above” option. Respondents can “opt-out” when none of the claims appeals to them. We believe this is very important, especially if the true aim is to find out which claim would trigger purchase.
But will results differ from MaxDiff? Not greatly. Across several tests we saw differences being within margin of error (and not differing substantially by method of calculating MaxDiff scores). Below is an example chart comparing preference scores for claims obtained through MaxDiff and through our methodology on a trial study on yoghurts (N=150, R2=0.92).
What does differ is the experience of respondents. Median length of interview: 5.4 minutes vs. 6.5 min on an equivalent test with MaxDiff (after removing speeders). We heard great feedback from respondents, and also observed higher survey satisfaction scores for the new Claims Test (4.4 out of 5 stars vs. 3.8 stars for the MaxDiff equivalent).
Claims Test scores are calculated using an analysis procedure called the Hierarchical Bayesian multinomial logit model. Preference scores are scale-less values assigned to claims to represent relative preference from each other. It is the same scale as partworth utilities in conjoint analysis.
Adaptive experimental design
One of the supposed benefits of MaxDiff is its ability to discriminate between items well. That is not disputed, but what we questioned was what it discriminates on: MaxDiff results (to simplify) add counts of “best” picks and subtract counts of “worst” picks for each claim. But when it comes to developing a winning product, the count of “worst” picks is much less relevant than count of “best” picks.
So we wanted a more relevant way to discriminate between claims. This led us to employ an adaptive experimental design algorithm (modified Thompson sampling). The algorithm listens to what claims respondents prefer and adapts the next set of questions (shown to other respondents) with the aim to clarify preferences around top claims (see Cavagnaro et al. 2009 for a deeper discussion).
The mathematics of this may be complicated, but outputs are not. Repeated tests of the same materials (with and without our adaptive design algorithm) show the ranking of top claims to be the same, except for greater certainty around top claims with the adaptive design algorithm. It is especially useful when testing fifty claims or more. To make use of this feature, select “Identify and zoom in on top claims” when choosing the goal of your experiment:
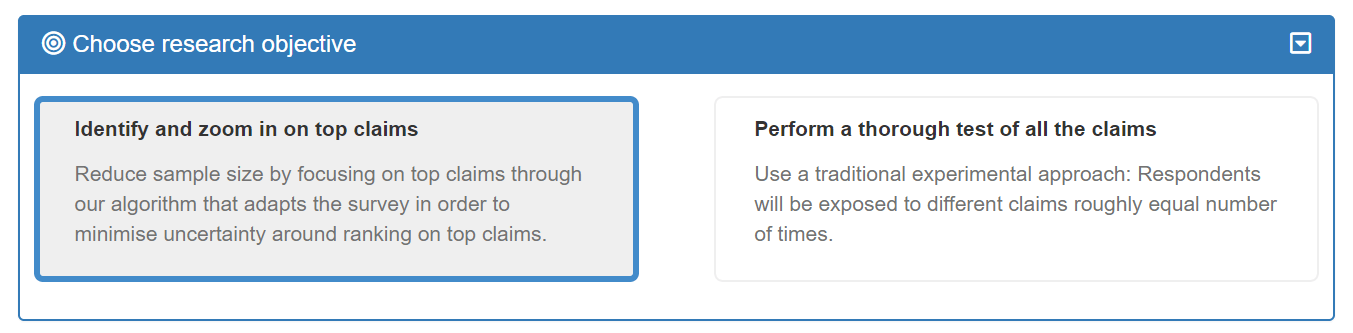
Allowing for cross-country comparisons
A pure MaxDiff does not answer another key question: Are any of the tested claims good enough? Both MaxDiff and choice-based questions will show a ranking of claims (relative to one another), but they will not show whether any of the claims hit the mark.
A potential solution is to supplement the study with Likert-scale questions. It’s a great approach, but we found time and again that rating grids are tiresome for respondents and hard to compare across countries because of acquiescence bias (the degree of which varies by country).
To combat this issue, we employ a well-known but underutilised technique: a dual negative-positive scale. For example, to diagnose a claim on relevance to consumer, we ask the question in two ways (each respondent will see only one of these questions):
- Give it this statement 👍👍👍👍👍 if it is relevant to you personally.
- Give it this statement 👎👎👎👎👎 if it is irrelevant to you.
With the help of a little arithmetic, scores are summarised in a digestible colour-coded table, which shows if a claim passed diagnostic questions and can qualify as a good one. Again, results are comparable with an equivalent study using rating scales (N=200, R2=0.65).