AI summary of open-ended text responses
With Conjointly’s AI summary of open-ended text responses functionality, you can automatically obtain high-level summaries and sentiment analyses for all open-ended questions in every report you interact with.
From $0.01 per summary, the function summarises open-ended responses to the following questions, including:
- Short text questions.
- Paragraph input questions.
- Positive/negative open-ended feedback questions.
- The ‘Other’ option for multiple choice and dropdown menu questions.
- Comments for highlighters in text highlighter and image heatmap questions.
- Open-ended diagnostics for Claims Test, Product Variant Selector, Brand-Price Trade-Off, and Market Test tools.
- Individuals’ commentary in Quick Feedback.
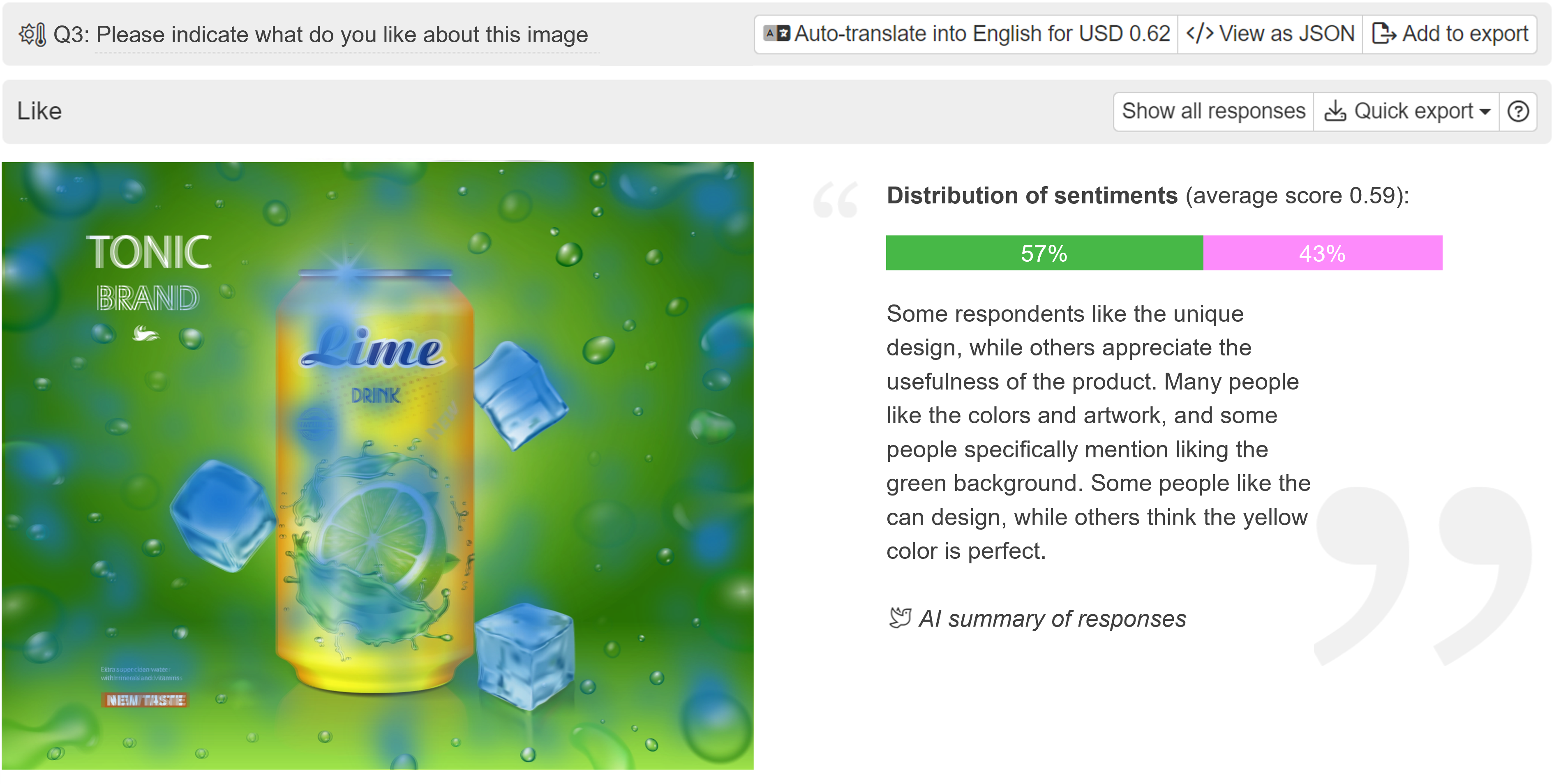
How to enable AI summary of open-ended text responses?
Here are two intuitive ways to enable AI summary of open-ended text responses once and for all your reports.
Method 1: Via Account Settings
On the left-hand side panel, click on Account settings under your login email to launch the menu.
Under the Auto processing of text-responses tab, check the tick box next to Auto-summarise text responses to enable it.
Method 2: Via Report Dashboard
In any report, the
button will appear on applicable sections and questions. Click the button to activate the function.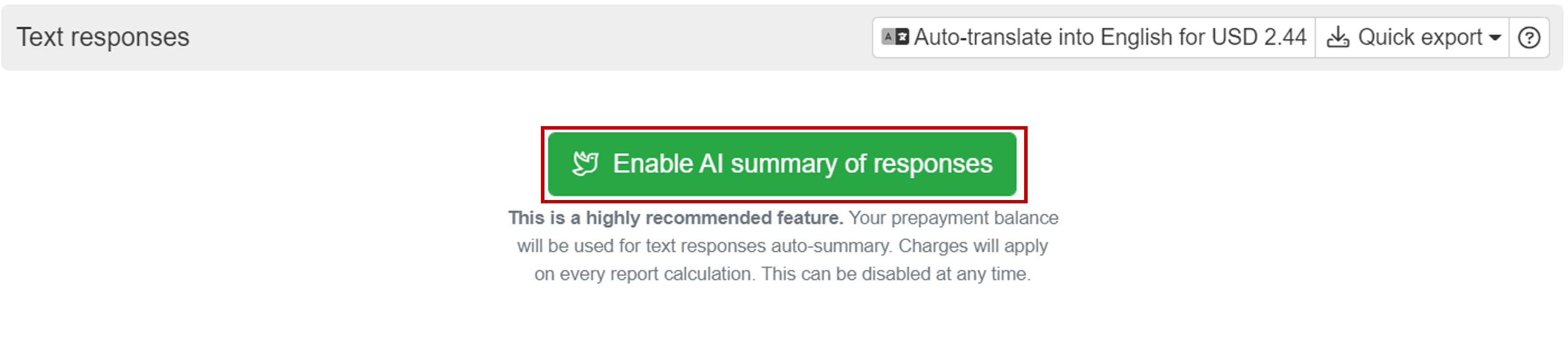
For comments in text highlighter and image heatmap questions, the button is located on the question tab.
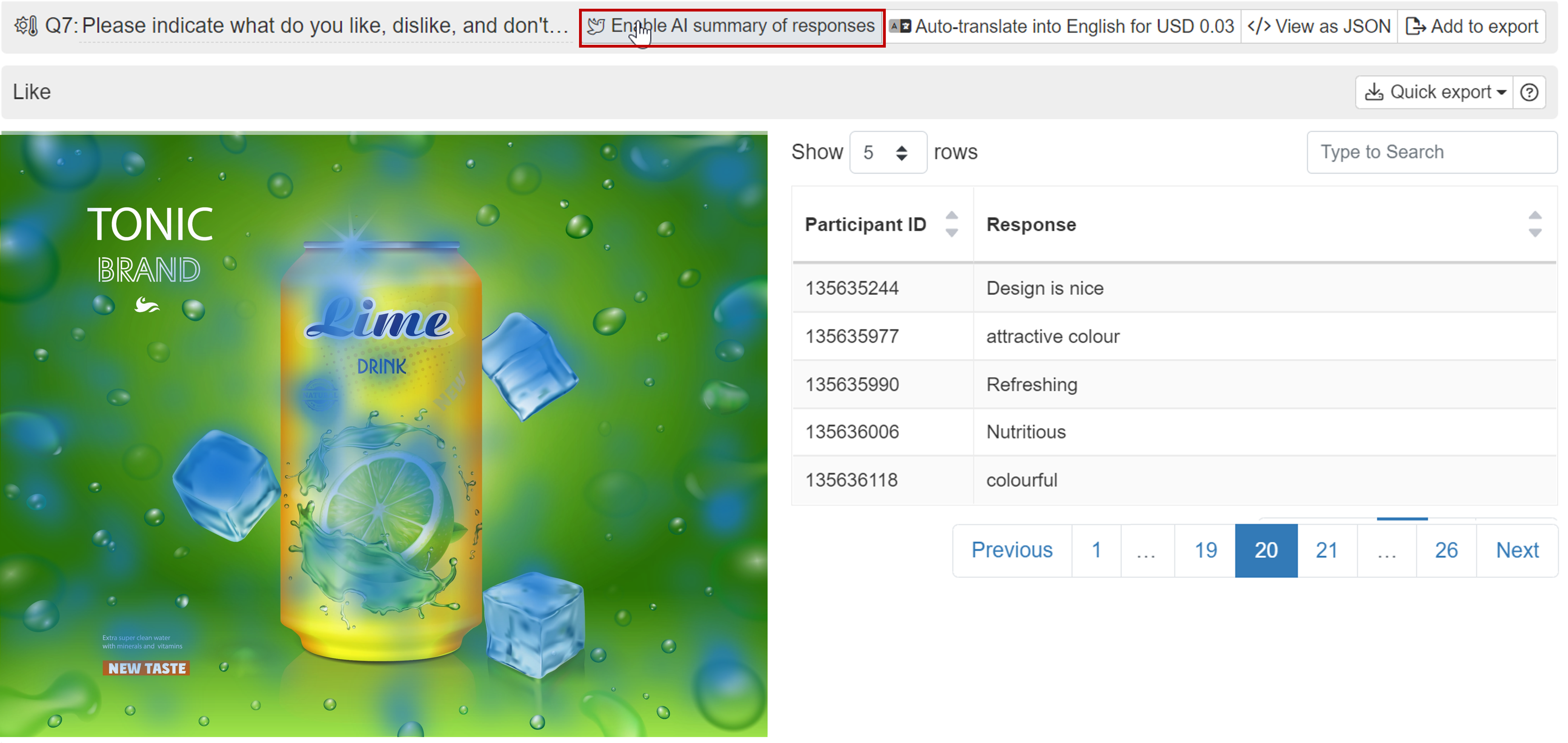
To enable AI summaries of open-ended text responses, you will be prompted to set up auto top-up if you haven’t already. Once enabled, your prepayment balance will be used for each AI summary of text responses. Charges will apply on every report calculation.
Sentiment analysis
Sentiment analysis helps you analyse respondents’ text responses to determine whether the dominant emotion is positive, negative, mixed, or neutral. The analysis is automatically included when performing an AI summary of text responses, and there are no additional charges for this functionality.
Interpreting sentiment analysis results for individual questions
For every open-ended question, the sentiment analysis outputs show the distribution of sentiments, which includes:
- The average sentiment score of responses, ranges from the most negative (-1) to the most positive (1).
- A coloured bar chart showing the percentage of responses by sentiment category, with green for positive sentiment, pink for negative sentiment, blue for neutral sentiment, and violet for the mixed sentiment.
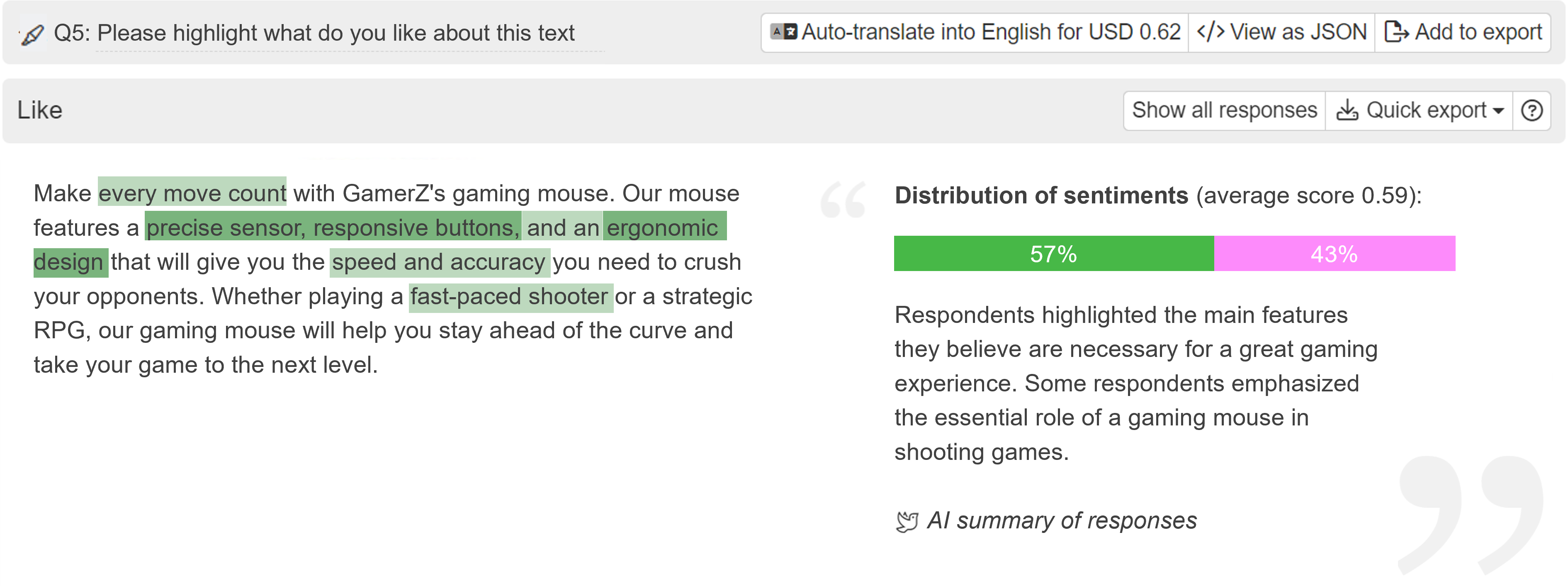
The following information is also displayed for individual responses to short text, paragraph input, positive/negative open-ended feedback, and the ‘Other’ option for multiple choice and dropdown menu questions:
- The prevailing sentiment determined by the natural language processing model
- The sentiment score, ranges from -1 (indicating a 100% likelihood that the response has a negative sentiment) to 1 (indicating a 100% likelihood that the response has positive sentiment).
You can also filter the responses by sentiment using the dropdown menu.
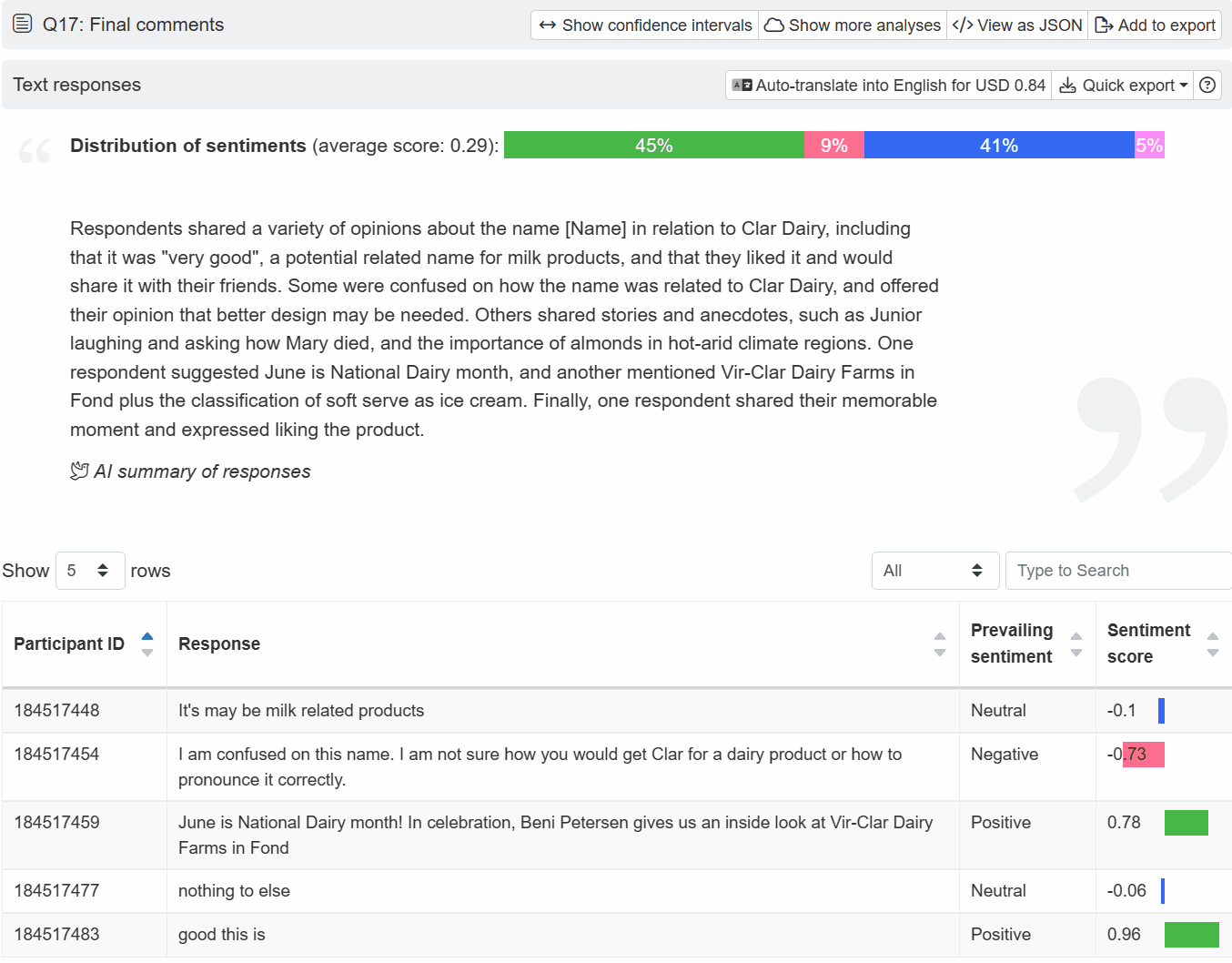
Interpreting sentiment analysis results for monadic blocks
For monadic blocks, the prevailing sentiment and the sentiment score are displayed for each stimulus in open-ended questions.
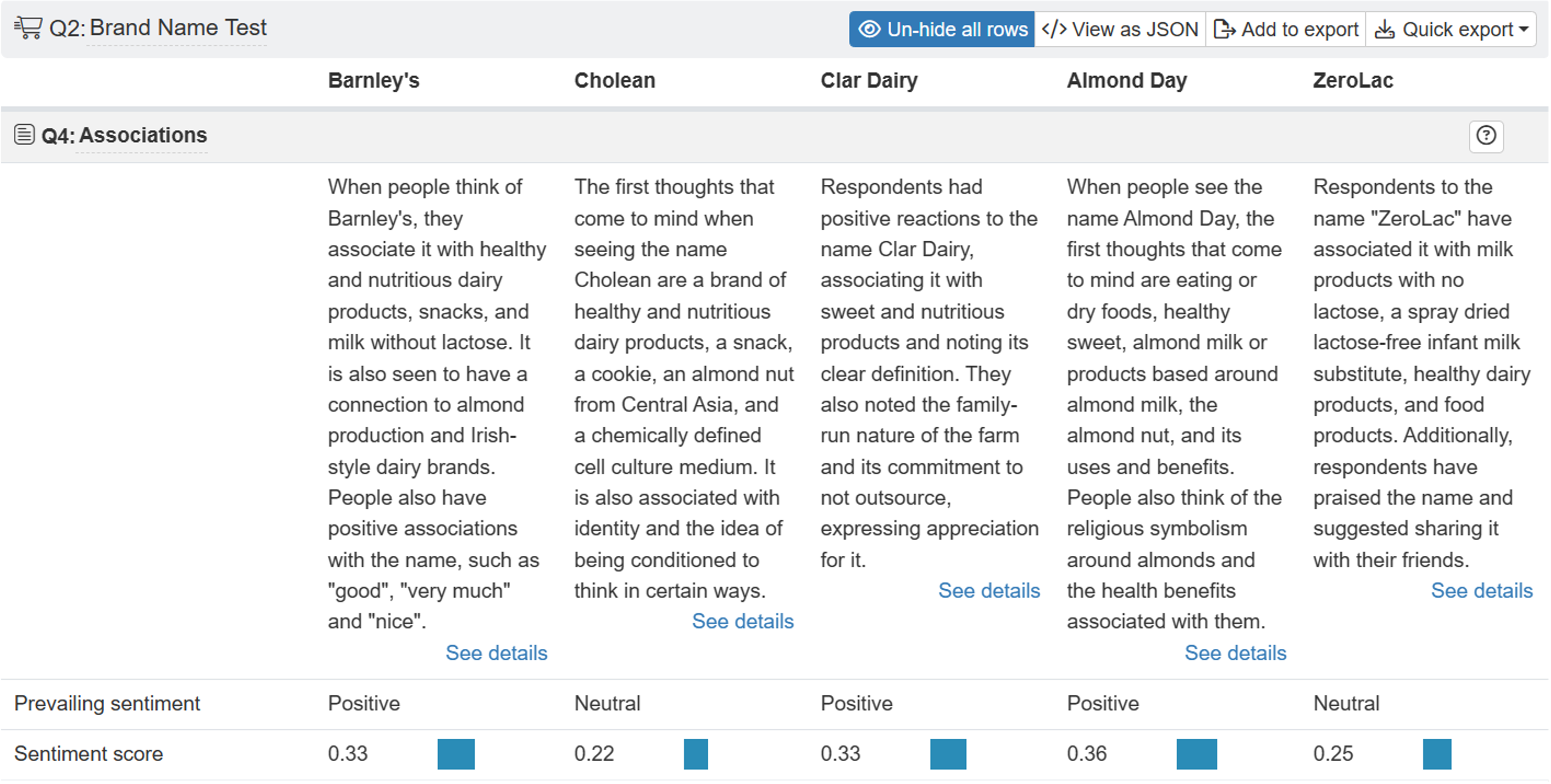
Using sentiment analysis results for segmentation
Follow these steps to create segmentation in your report using sentiment analysis results.
- In your report, navigate to Segmentation .
- Click on .
- On the pop-up, select .
- Specify the question, item (only if applicable), operator, and sentiment from the dropdown menus.
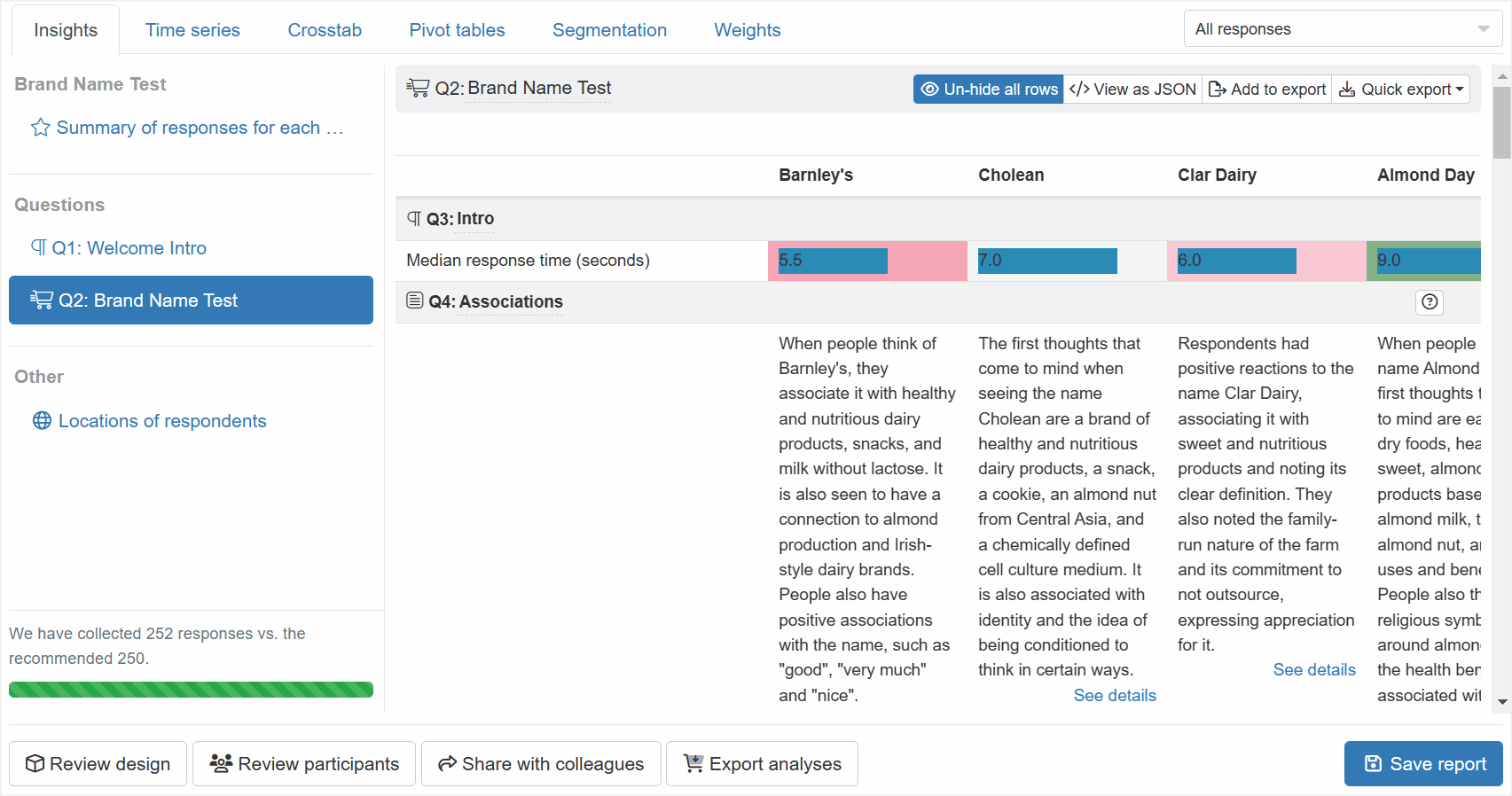
FAQs
How do AI summaries of open-ended text responses work?
The AI summaries of open-ended text responses are powered by the GPT-3 natural language processing (NLP) model. All responses are preprocessed to remove answers that do not contain valuable information, such as “Nothing”, “N/A”, and empty answers. Then, the AI-based model processes and summarises the content.
While models like GPT-3 are a great help in working on natural language tasks (that would otherwise require manual human work), it is not always clear exactly why the model provides a certain output. By design, AI’s exact way of “thinking” is not always clear and is a deep research field.
Conjointly encourages treating AI summaries as high-level summaries that take into consideration all text inputs with valuable information.
How are AI summaries of responses priced?
Why do I need to set up auto top-up to enable AI summaries of open-ended text responses?
How can I disable the AI summaries of open-ended text responses?
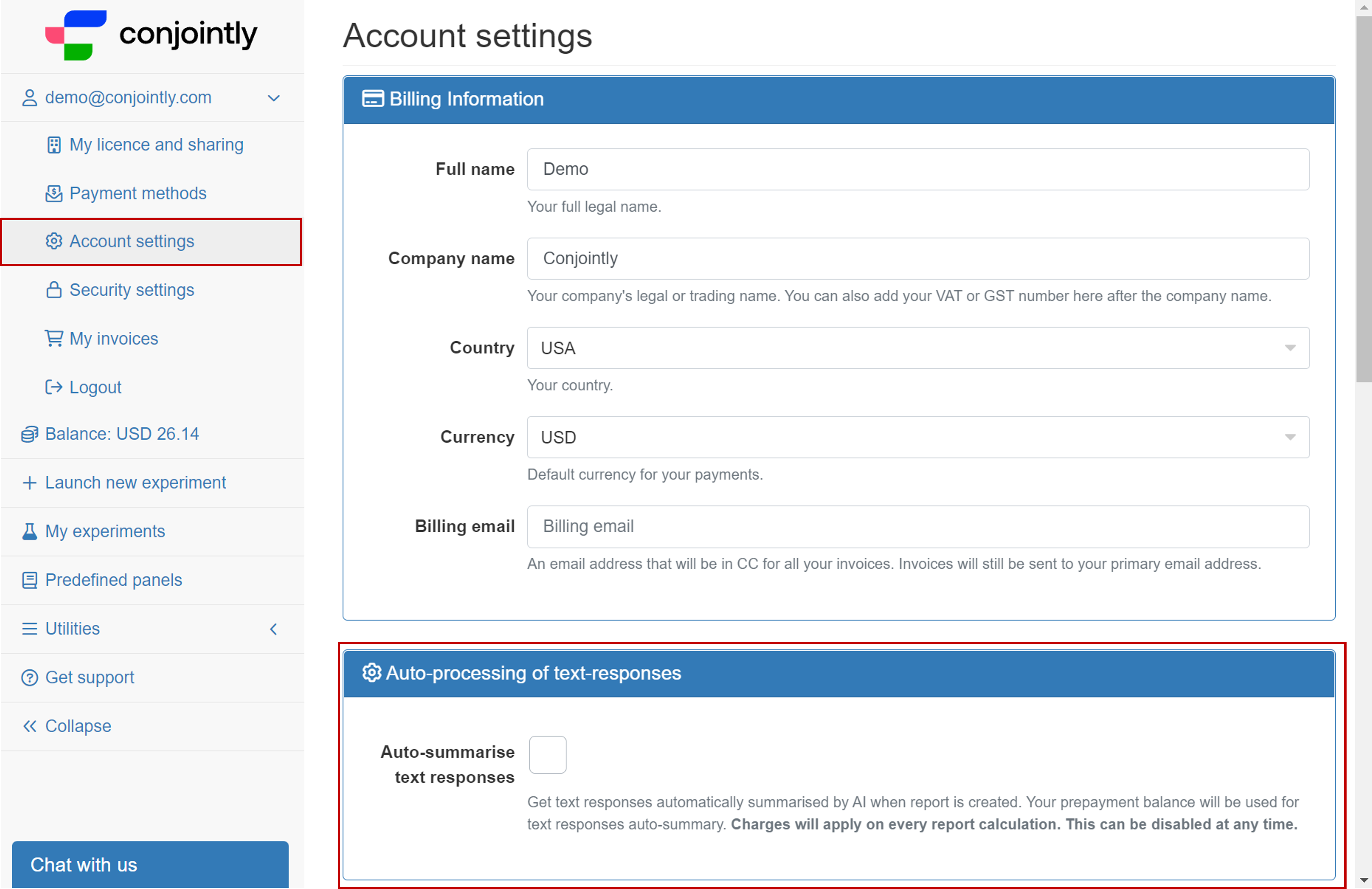